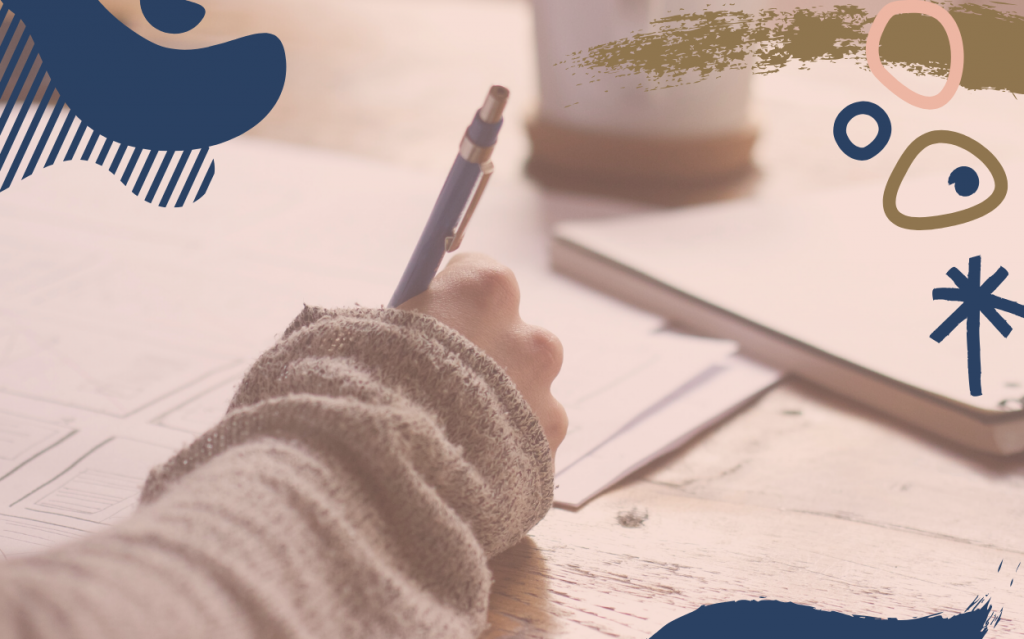
Until as recently as 5 years ago, text data was considered the little brother of structured data. Consumer insights were mainly based on market research surveys, which often contained several closed-ended questions and one open-ended question. Responses to open questions were coded manually, and insights were mainly derived from structured questions, using the coded open answers to (hopefully) confirm those insights. However, times are changing.
The old way of looking at text data is growing obsolete
Data is changing: the digital revolution has led to a vast increase in the amount of text data in existence. Words are all around us. Social media, customer service centers, web forms, forums, emails, surveys, the list goes on: so much talk is generated about your brands, products or services across a wide range of channels. The amount of text available often excludes manual coding as an option for analysis, as it is too expensive and time-consuming – and not even very accurate.
The best approach to uncovering text insights
At Boobook, clients often ask us how to reveal the wisdom behind their text data. The easy answer to this question: text analytics. However, text analytics can take many forms, and choosing which approach to choose is not a walk in the park. Choosing the right approach depends on different specifications, such as the nature of the source, client needs, data volume, languages used and reporting frequency. An experienced analyst is indispensable in guiding you towards the ideal solution.
4 main approaches to text data analysis:
1. Word counting
The easiest approach is based on word frequency. Visualizations in the form of word clouds offer initial insights into, for example, what people are saying about your brand. Even a ‘simple’ word cloud can become interesting if we link the words to structured data. You can ask these questions:
- Do my prospects say other things than my clients?
- What do my satisfied and dissatisfied clients say about my product?
- Does my brand clearly differentiate from my competitors?
2. Theme identification & sentiment analysis
With text analytics, we can automatically code text into themes and even determine the sentiment of the text (positive, neutral or negative) without a Net-Promoter-Score or an extra question concerning satisfaction. Different levels of human involvement are possible to identify the themes:
- Human involvement is lowest when applying ‘topic modeling’. This analysis reveals how words are linked together and allow messages to be grouped into themes, a process partly supervised by the analyst to ensure themes that make sense. Many different models exist, such as probabilistic, deterministic, and several others. However, the result is the same: the computer performs most of your coding work.
- If you have specific themes you want to code the data into, a small portion of the data is coded manually. This partial coding forms the basis of a model that codes all other data via predictive modeling, a process based on machine learning.
With both approaches, we can apply the same coding rule when new data comes in. These methods minimize coder bias (especially topic modeling) and guarantee comparable coding between waves.
3. Outcome prediction via machine learning
We can even take text data analytics a step further and predict specific outcomes. Some examples:
- Use online reviews to uncover which words/concepts trigger good or bad reviews. This model can be used to predict customer satisfaction based on Twitter or Facebook posts. It allows you to filter on negative comments, eliminating the need to go through all comments every day. Focus only on the ones that need follow-up.
- Based on customer service responses, we can predict churn and prioritize customers for intervention.
4. Interpretation & feedback via text analytics
With natural language processing (NLP), grammar and sentence structure also comes into play. NLP allows for a deeper understanding of the language, where words are categorized as nouns, verbs, adjectives, and so on. This information can be used to support the analysis and to add an extra level of context. This technique is mainly applied when you need a responsive model that can interpret questions and give feedback. Nowadays, smart chatbots can recognize human language through natural language processing (NLP) and generate a response.
Want to know more?
Text data analytics is a very large container that answers different needs, from the creation of a word cloud to the prediction of a specific future behavior. Each approach has its advantages and its pitfalls. Here at Boobook, we are happy to help you choose the best approach to deriving true wisdom from your text.
Related posts

Generational marketing: How to connect with Gen Z
We’re continuing the series on generational segmentation with Generation Y or Millennials, individuals born between 1977-1995.
