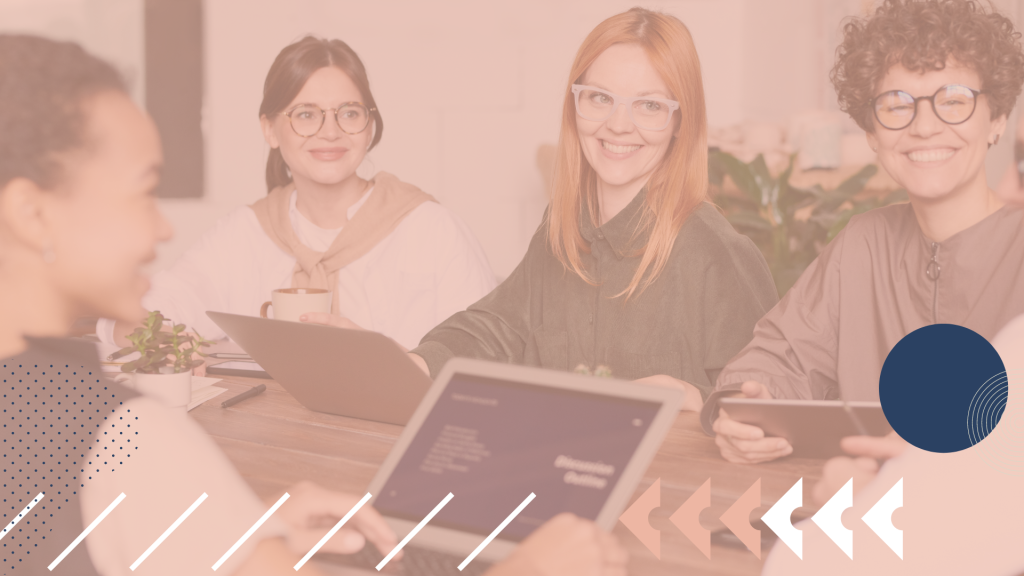
In our previous article, we introduced a 10-step guide on setting up, developing, and finalising a successful customer segmentation. We continue our series with an overview of segmentation tools and methodologies to help you efficiently and accurately carry out your market segmentation.
The main objective of intelligent customer segmentation tools is to help you automate your segmentation to access and visualise data from multiple sources to gain essential insights and optimise your marketing and sales.
A segmentation tool is a great way to personalise each user's experience and make it unique - for them. Thanks to continuous innovation and growing interest in customer insights, there are many tools available on the market today. So, if you're wondering which customer segmentation tool would be the best solution for your business, here are the top 5 customer segmentation dashboards and analytics tools we recommend:
Google Analytics is the most common tool used by almost every business out there. Google Analytics is a popular option among marketers because it is free and pulls out all website traffic data. Thanks to its robust features, you can do pretty much anything, from tracking your eCommerce funnel to developing customer segments and retargeting website visitors or customers. Even though it's a standard platform, Google Analytics still requires specific knowledge to get the most out of it.
Segment collects your customer data in one place and sends it anywhere. This is helpful for organisations that want to enforce the same data standard across their enterprise. You can then power all your apps with the same data. Using this customer segmentation tool, you can query your data in SQL for the most convenient experience. The segment also offers Personas, a new product built on the Segment platform. With Personas, you can make sense of your customer data and deliver personal experiences throughout the customer journey.
Hull's unique customer data platform unifies your data across your stack, unlike other solutions that create one-to-one integrations from one application to another. Hull gives marketers more visibility, control, and flexibility over their customer data to execute their ABM and demand generation initiatives effectively. Their real-time, 2-way synchronisation capabilities allow the data in your tools to stay up-to-date, so your teams remain aligned.
Kissmetrics is an extremely popular analytics dashboard that integrates with most eCommerce websites and digital marketing tools to centralise data into a single dashboard to create audiences and customer segments that support CRM systems and digital marketing campaigns. Kissmetrics is focused entirely on tracking users through the customer lifecycle. It provides insights about customer lifetime value (CLV), free trial conversions, and similar behavioural triggers that help your business grow.
Adobe Analytics creates a holistic view of your business to truly understand your customer's journey on the web. The platform enables you to track your digital marketing campaigns and their performances, recognise and create different types of data segments for remarketing, offer a customised shopping experience to your site visitors, and work with bare-minimum data latency, particularly during periods of peak traffic activity. Adobe Analytics Cloud also connects to a wide range of native and 3rd party tools to quickly bring in 3rd party data to the tool.
- Social Media Platforms
Social media platforms like LinkedIn, Facebook, Instagram, Tik Tok and Twitter are all packed with helpful features that help businesses segment their audience and target them with paid ads. Segmentation with social media tools can help you break down and organise your target audiences based on shared characteristics like interests, job function, demographic information, behavioural habits, and geographic location.
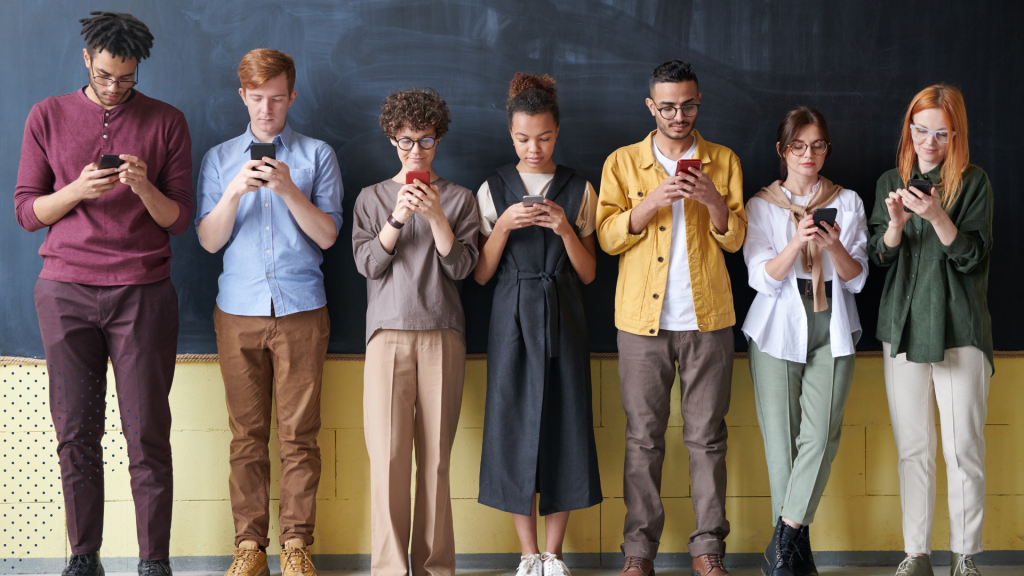
Traditional vs Cluster segmentation
As you set up expectations and objectives for your market segmentation, the next step is to plan the data environment for the segmentation methodology. Each method requires a different set of information. For example, strategic segmentation requires a long history of financial consumer data, looking at what products or services the consumer purchased, how much was spent, and how long. Marketing-focused segmentation (behaviour, needs, and lifestyle) requires a more detailed set of customer data, such as what products or services the customer uses, the motives behind this use, the customer's response to campaigns, complaint data, and the customer's perception of the brand.
Before diving into each methodology separately, we should emphasise the difference between so-called traditional and cluster segmentation.
Traditional market segmentation research is often used to identify and differentiate various segments in terms of:
- Purchase decision criteria
- Benefits desired/customer needs
- Category behaviour and attitudes
- Brand/corporate attitudes
- Lifestyle/psychographics
- Demographics
While this model of segmenting puts customers into groups based on similarities, clustering is a process of finding similarities in customers. Clustering is the predictive marketing version of segmenting that can help us foresee what customers are likely to do without putting them into rigid groups.
Thanks to cluster ensembles, researchers developed robust and reproducible segmentation solutions based on both multiple batteries of questions and alternate clustering algorithms. Cluster ensembles employ a two-phase process: phase 1, where we develop multiple cluster analyses that vary in terms of the clustering method employed, and in phase 2, clusters and groups respondents based on the cluster analyses generated in phase 1.
Overview of methodologies
- K-means Cluster Analysis: K-means clustering is a method of vector quantization, originally from signal processing, that aims to partition n observations into k clusters in which each observation belongs to the cluster with the nearest mean (cluster centers or cluster centroid), serving as a prototype of the cluster.
- Latent Class: One of the most common—and one of the trickiest—challenges in data analysis is deciding how to include multiple predictors in a model, especially when they’re related to each other. LCA is a measurement model in which individuals can be classified into mutually exclusive and exhaustive types, or latent classes, based on their pattern of answers on a set of categorical indicator variables.
- Ensemble: In the context of market research, Ensemble Segmentation may mean combining segmentation solutions that have been developed independently from behavioural and attitudinal data on the same sample, thereby increasing our understanding of customers. In the context of CRM data, this may mean combining solutions that have been developed independently using marketing data and sales data, thereby allowing us to add customer intelligence to sales activity.
- Decision tree, e.g. CHAID: CHAID is a type of decision tree technique based upon significance testing. It can be used to create rules to classify future respondents into identified groups using several different questions or to detect interrelationships between different questions. For example, the combination of age and gender helps to further explain the different types of snacks that people consume.
- Discriminant analysis: Discriminant analysis builds a predictive model for group membership. The model is composed of a discriminant function (or, for more than two groups, a set of discriminant functions) based on linear combinations of the predictor variables that provide the best discrimination between the groups.
- Multinomial logit (MNL): Multinomial logistic regression is used to predict categorical placement in or the probability of category membership on a dependent variable based on multiple independent variables. The independent variables can be either dichotomous (i.e., binary) or continuous (i.e., interval or ratio in scale).
- Random Forest: Random forests or random decision forests are an ensemble learning method for classification, regression and other tasks that operates by constructing many decision trees at training time. For classification tasks, the output of the random forest is the class selected by most trees. For regression tasks, the mean or average prediction of the individual trees is returned.
If you need help or guidance with customer segmentation, feel free to reach out! Our team of experts will help you expand your knowledge about your customers and provide tailored solutions based on in-depth analysis and insights.